In this episode of the AI For All Podcast, Tom Andriola, Vice Chancellor and Chief Digital Officer at UC Irvine, joins Ryan Chacon and Neil Sahota, to discuss the impact of AI in universities and the workplace. The conversation covers how AI tools can transform education and business operations, managing unstructured data, how AI has evolved over the years, and how companies can leverage AI. They also discuss the future of AI in workplace scenarios, where AI could lead in the next decade, and the importance of making AI accessible for all.
About Tom Andriola
Tom Andriola is a business and technology executive who has been at the forefront of technology-enabled transformation for three decades. He currently serves as UC Irvine’s Vice Chancellor and Chief Digital Officer, ensuring the strategic use of data and technology transform the mission of the modern university and the future of health. He has been an innovator throughout his career, which has spanned across the public and private sector and included running tech businesses on four continents.
Interested in connecting with Tom? Reach out on LinkedIn!
About UC Irvine
Founded in 1965, UC Irvine is a member of the prestigious Association of American Universities and is ranked among the nation’s top 10 public universities by U.S. News & World Report. The campus has produced five Nobel laureates and is known for its academic achievement and premier research and innovation. UC Irvine has more than 36,000 students and offers 224 degree programs. It’s located in one of the world’s safest and most economically vibrant communities and is Orange County’s second-largest employer, contributing $7 billion annually to the local economy and $8 billion statewide.
Key Questions and Topics from This Episode:
(00:31) Introduction to Tom Andriola and UC Irvine
(02:31) AI in universities
(03:53) Impact of AI on healthcare and research
(04:34) Challenges and approaches in AI implementation
(08:28) Making AI available to all
(10:12) AI in the workplace
(16:17) AI interpretability and interoperability
(21:16) Role of AI in business decision-making
(25:47) Future of AI and the metaverse
(33:54) Learn more and follow up
(02:31) AI in universities
(03:53) Impact of AI on healthcare and research
(04:34) Challenges and approaches in AI implementation
(08:28) Making AI available to all
(10:12) AI in the workplace
(16:17) AI interpretability and interoperability
(21:16) Role of AI in business decision-making
(25:47) Future of AI and the metaverse
(33:54) Learn more and follow up
Transcript:
- [Ryan] Welcome everybody to another episode of the AI For All Podcast. I'm Ryan Chacon and with me today is my co-host, Neil Sahota, the AI Advisor to the UN and founder of AI for Good. Neil, how's it going?
- [Neil] Hey, I'm stoked, Ryan. I'm actually really looking forward to our episode today because it's a near and dear topic to my heart, which is education and how AI is a tool to help transform that experience.
- [Ryan] Yeah, absolutely. So, we got a lot that we're going to cover today. We'll talk about that. We'll talk about AI's impact in the workspace itself. But before we jump into our conversation, I do want to introduce our great guest, Tom Andriola, the Vice Chancellor and Chief Digital Officer at UC Irvine. Tom, thanks for being on the podcast.
- [Tom] Thanks for having me, Ryan.
- [Ryan] Yeah, it's great to have you. Let's kick this off and just learn a little bit more about your background experience and tell us what a Chief Digital Officer does at a university.
- [Tom] My background is an interesting blend. One, early stage of my career in the consulting world. Then I jumped over and was a Chief Information Officer. I like to refer to myself as a recovering Chief Information Officer. Was then able to do mergers and acquisitions and learned a lot about integrating companies and putting business operations together on a global level. Then became a general manager of software businesses, the combination of understanding how businesses work and understanding the technology space, started then running software companies when in my years working with Philips, a very large global company.
And then about 10 years ago, I jumped over to the university world. So a lot of that experience in the corporate world was in the healthcare sector. So the software and software as a service platforms were for healthcare customers. Came to the University of California, where we do a lot of healthcare, but we also do education research, which is probably what people think of first. And so I had to learn a new sector. In addition to continuing to serve the healthcare industry and be a thought leader there, really have learned a lot about how does education work, how does sponsored research work? And then what is it like to be a publicly funded organization? And blending kind of private sector and, private and public sector thinking for problem solving, right, because our really complex problems require the public and private sector partners to work together in ways that we maybe haven't in the past.
So it's been an interesting journey, had a chance in those different experiences to work and live on four different continents. And so I have this kind of perspective of the world of having seen it at a very global level, but then also seeing it at a very local level, which I appreciate.
- [Ryan] Yeah, that's fantastic. So how's, how are you seeing AI being used, before we get into the workplace conversation, which I know is a big part of what we want to talk about today, but just in the university setting, how are you seeing AI kind of play a role? Or how's it being, is it more so focused on how it's being taught in the, to students? Like where are you interacting with AI in the job that you do?
- [Tom] Yeah, I think it's touching everything. I think if you talk to most organizations, they're talking about a kind of a seminal moment where there's going to be an inflection point, much in the way the internet created an inflection point for businesses a generation ago. I think a lot of us, and again, it's not just if you're a technology leader, but if you understand new tools that create inflection points, you see this as one of those seminal moments.
So if I step into the university world, let's say the research and education side, there's going to be a tremendous impacting in the way teaching and pedagogy works. There's going to be a tremendous way that we use this tool for the way that we actually run a campus. And running a campus is like running a small city. With 37,000 students, 20,000 employees, it's, you're running a small city, and so it's going to transform our thinking around that. It's, these new tools are already transforming the way that we think about pushing the boundaries of discovery and research, how we interact with collaboration partners, be them other universities or the private sector. And if I jump over to the healthcare world, it's changing already the way that we interact with patients and the way that we think about patient care.
I think it's going to create a lot of change. But it's a long game. I say it's like the Tour de France. We're in the first stage. OpenAI may have won the first stage and have the green shirt, but it's a long game, and I think you're going to see a lot of twists and turns on the way that this gets used, who emerges as real, with real differentiation around using it. And so I'm just excited for the next several years.
- [Neil] A lot of organizations today, I hear the same question. We know we should be doing something with AI, we just don't know what it is. And you have worked with a lot of diverse stakeholders, the researchers, educators, the students, alumni, workforce transformation, all these things, and you've actually put together a lot of great projects. I know you just launched ZotGPT. I think everyone's just wondering like how did you do that? What was your approach to try to figure this out?
- [Tom] There's one constituency that you didn't mention, which is from my platform as Chief Digital Officer, I also get to work with the private sector a lot. And so we had last Fall an event that we hold annually with the business community. So these are usually large, multinational companies, think the Hitachis and the HPs of the world and the Targets of the world, and I get a chance to listen to them talk for two days about what they were doing because they tend to move a little faster on this stuff than we do. And it really informed a certain approachable institutionally, but I also believe that there's an individual journey for each and every one of us. And let me start there, right?
So I think every one of us with respect to having this new tool or set of tools available to us has to go through a cycle that goes something like this. I've got to investigate it. I got to experiment with it. I got to learn it. I got to do some early kind of productivity hacking my own world with it. And then I figure out how I could use this in the broader business context and then how to scale it. I don't think you can skip any of those steps. I really don't. And so part of you saying why did you launch ZotGPT is to get more people into that cycle because I don't think you can have a work re-imagined initiative that we have without a large percentage of your population getting over their initial fear and anxiety around these tools.
And so like when I get in front of audiences that are a little bit more on that side of the continuum, I'll say to them, look, here's a really easy first thing to go do. You all do Google searches. You could flip one button, and you can now get your Google search alongside a GPT type summary and just look at the results that come from those two things and then ask yourself, do I want to figure out which five links that I want to click into to quote unquote go through the cognitive exercise of putting together a summary, or do I want to take what that summary that was just written for me? I said just ask yourself that question. Just to familiarize yourself with what can these tools do today? And then what do you think they're going to be doing a year from now? Anybody can do that. And so people come up and saying that was actually really pragmatic. I'm going to try it, I'm going to start doing that. And now it's really interesting to see how people evolve from that to now seeing things.
What did ChatGPT do for us? One, it gave people that it's okay to play, and the big, and it also addresses the biggest fear that was expressed to me, which is if I put my data in this, do I lose it to the OpenAIs of the world, the Microsofts of the world, and so we put a wrap around and said, no, you don't have to worry about that. What you put in here, you don't have to worry about that going into the public domain if that's data that you care about. And then we explain to people how that works.
So the initial launch is really much more about getting people onto the playing field. As we get more people on the playing field, what we're seeing is the emergence of those early use cases where we can create better outcomes are now starting to emerge. And then we're parlaying them back into share your story with others because when they hear your story, they're now going to have a better idea of what they might be able to do.
- [Neil] That's awesome. I think that's advice most organizations can actually put to use. I like the fact you addressed the people challenge because I think that's where I see a lot of kind of stumbling around is the antsiness and the jitteriness around the technology and what's it going to do? Is it going to make a mistake? Do I get liable for that?
- [Tom] You started an organization called AI for Good, right? Really trying to focus on the positives. I was on a call earlier this week, and we were also talking about AI for all. In some of what's happening, and we were talking about a particular movement that's happening to try to make sure that AI is brought in in a responsible way, but in some of the governance around who's going to sit at the table and help make some of those decisions, create some of those structures, there are a lot of different voices and elements left out. I'm trying to be a little bit obscure here. One of the things, especially being with a public institution is AI for all, right? And I brought up the point yesterday because our ZotGPT is initially launched just for faculty and staff. And of course I'm getting the question what about students? And there are some really good reasons why we want to slow walk towards giving it to all students. But I'm one of the ones saying sooner versus later for equity reasons because the students who are using it right now are either using an inferior version that's available in the marketplace or able to pay the $20 per month to get access to the latest model.
That's an equity issue for an organization like ours. We need to level that playing field, or we're going to have our own version of the digital divide or societal disparities that we've created around the next wave of technology. And I'm one of the voices saying we can't do that. We got to do a better job this time and not go through what we just experienced with the digital divide of the internet for the last 20 years.
- [Ryan] Based on everything we talked about, I'm curious if we talk a little bit more about the wider group of organizations that you've had experience with, interacted with, and seen AI impact over your career. How has AI impacted the workplace over, say, how's it changed from maybe five years ago to where it is today? And what are some of the struggles that companies are having to really leverage this technology just across the board?
- [Tom] Yeah. So I think if you go back five years ago, you're now talking about just pre pandemic. We're in 2024. You're talking 2019. When we talked about AI, and I always like to say, so when you, if you say AI, what do you really mean? And so back then, AI for a lot of people was really about machine learning, using machine learning algorithm type things for predictive purposes. We were dealing a lot with structured data and when we were talking about unstructured data, we were talking about natural language processing to try to figure out how to pull out the right types of elements out of unstructured data and put it into a structured format, so we could use it in our machine learning models.
And that's really, that's where we were. But the introduction of generative AI opens up for, I think, all organizations, and I'll give you an example in a second, to use all of their data. So if you look at a healthcare enterprise, a health delivery organization, which we are one, most statistics would say three to five percent of the data is structured. The other 95 to 97 percent is in an unstructured form, means it's some types of textual blobs or unstructured type formats. So that means in 2019, most of our analytical models that we were using, whether they were traditional or advanced analytics, were only leveraging 3 to 5 percent of the entire data, digital information that we were holding as an enterprise.
So, you got to think about that. We talk about patient outcomes, right? Or same could be said about our student success initiative in terms of how we're using analytics to help support students reaching their academic and career readiness goals. Well now all of a sudden we can open up to the other 95 percent of data. That is incredibly powerful if you think about it. And I think that's what one of the big things that's different is we were very limited in the type of data that we thought we could use and generate benefit from, and we had these limitations, and those limitations have been completely lifted. And now what you're talking about is how, do I even understand all the digital information that I have? How do I get it into buckets where I can put these models on top of it and start churning out answers, right, or outcomes? How do I know whether these, those outcomes are the right types of outcomes, whether they're equitable outcomes, whether they're responsible outcomes? So do I understand my data enough to understand about the ability to hallucinate or the ability to manage hallucinations?
So the world has changed I think a lot. We're dealing with now I think magnitudes more of data available for these types of analytical frameworks and predictive algorithms. And I think we're learning with this like those that actually had really good data management strategies actually have a differentiated advantage to move faster in this now world of I like to call it augmented intelligence. AI is augmented intelligence because I think we're very far away from automated decision making, and I think we're much closer to human in the loop type decision making, but now using better, more robust information to inform an individual to make the final decision.
- [Ryan] Yeah, that data management that you mentioned I think is really important because we've talked about in the past about how can organizations not only get good data and access to data, but these companies that have lots of data, what, how can they manage it properly to be utilized by new technologies, new tools, to help them make better decisions. And I think that's a really big challenge for a lot of organizations. Once they get the data is what, how can they manage and what can they do with it? But even if you take a step further, back by that, there are a lot of companies that listen to this that don't really know what kind of data they have access to or are unsure how to get data that is going to be useful when they're able to bring in a tool to better analyze it with the advances of AI and things like that as well.
- [Tom] And the whole digital movement, there's more and more of our world that is being captured now with a digital signal. And if that what that digital signal is more data. So, I use the example, a few months ago, I was talking about basically how do we support students to succeed in a much more kind of holistic and personalized way at our university. And then the last slide I use with the audience, because we're talking about the classroom, learning data. We were talking about everything we know about their enrollment. We were talking about student life and all their activities and career readiness, their internships, their summer jobs, their competency building. And then my last slide was the first metaverse class that we taught at the university. And I said we're teaching a metaverse class and the data that's generated from students being in the metaverse, what they interact with, how long they stay, where they portal to, what their eye movements are, this is all now new data. I doubt it's going to be well structured. But it's going to have to find a path into the, where the rest of our student analytics data sits, and we're going to have to figure out what type of insights we can generate from it. And I just use that as an example of saying there's constantly new data coming online for us that we have to figure out how do you bring it into a usable form and combine it with the other data that you've already aggregated and curated together. And so you've got to build a mechanism to be able to do that and have it be actually quite rapid and agile because that's how fast the digital world is moving for us.
- [Neil] To be a successful business, you have to move at the speed of technology, right? But I think to your point, Tom, that's a big challenge because we're collecting lots of data. We're discovering new variables and data, and we have struggle keeping up with that. I brought this up in a conversation this morning where we were talking about this traditional analysis, you looked at six or seven variables, but now we have the ability to collect so much more data, especially around behavior and psychographics that now there's 200 relevant variables, so the only way they can do this is with AI.
But I think this alludes to some of the challenges you were talking about earlier, that we've always talked about two big challenges, which was interpretability and interoperability. Interpretability for the audience is the AI comes up with some sort of answer, recommendation, but the business or domain experts don't quite know where that came from. And everyone goes that's fine, the technologists who built it understand how that came to be. But that's actually the interoperability issue that we're seeing more and more that the technologists don't know how that conclusion was arrived at. It's too much of a black box, or it's just too convoluted for us as human beings to be able to track that many variables.
But I think there's a third one now, which you started alluding to Tom, which is prompt engineering. That you talked about hallucinations. We're starting to see that poorly constructed prompts or missing parameters from your prompts causes problems. Like we've all heard the story about, I think it's happened a dozen times now, the lawyer goes into court, had ChatGPT or something like that research the cases it's going to cite, and it made it up, hallucinated. And I actually dug into this and the problem was the prompt. They're like give me three or four cases that support my case strategy. So, gen AI doesn't know you need real cases. So, it's just like cool, I'll make some up. They're perfect fits for you, right? Now, shame on the lawyers for not doing the validation, but I think that's what we're starting to see more and more of is that it's about prompt engineering, but I think we're trying to learn how some of these things work, the parameters to it. We don't actually fully understand that. Is that something you're starting to see, Tom?
- [Tom] I love your example. I think you're spot on. I was in a discussion with a company that we work with, and it was with basically their leader of software development. And we got into a whole conversation about, they've now plugged their model into one of the large language models, and I'm like how does that work? Walk me through the interactions in your software, the call, the come back. And essentially at the end of his description, I said, you're working towards the perfect prompt. The quality of your answer ultimately comes down to the curation of the data that we've given you, your ability to use a large language model, but what you're really trying to generate is the perfect prompt to give the best contextualized answer back to the requester.
And then I was very interested to get some comments about how much is that costing him to generate that perfect prompt, ping back and forth. But it was, it really brought home your point, which is it ultimately comes down, the quality of the prompt will determine the quality and the accuracy of the answer. And also, and his point to me was how contextualized the answer is. Moving from the earlier generation technology where if they didn't ask the question the right way, it was going to tell you it didn't have an answer. Now, if you ask the question, you have to understand the context of the question to provide the best answer, else you're going to get the second iteration, the third iteration, which is going to frustrate the end user. And I thought it was fascinating. And this is a relatively small software company, pretty small number of use cases. I would love to see what a large software company, like a SAP or an Oracle or a Salesforce is trying to think about with this stuff because they're just so massive in terms of what they have and what they do. I can't imagine, I would love to get inside and understand how they're trying to make this stuff work because that's where we're going to see a lot of this, through the tools that we've already deployed in our enterprise. Everybody's got their story about, Salesforce, it's Einstein is what you're going to bring into it. And for this company, it's something else. And Microsoft, it's Copilot. And so everybody's got their story, and that's where we're going to see a lot of and quite honestly, if we don't ask questions, it's going to be a black box.
- [Neil] I've seen a lot more people now, especially domain experts, start to realize that they don't fully understand how some of the stuff they do works.
- [Tom] Luckily, we have students, Neil, because that's where I go when I'm trying to understand how it works. I reverse mentor this thing.
- [Neil] That's a great endorsement of why you have to hire students, particularly, especially when they graduate. Fresh ideas. They don't come with the legacy infrastructure limitations.
- [Ryan] Tom, let me ask you, we've been talking about some good topics here, but if I'm listening to this and trying to understand and prepare my organization to leverage AI in the workplace, what advice do you have for those companies on how best to approach it? What should they be doing about analyzing the data they have access to, evaluating the data is good or not, collecting more data, and then how can they evaluate what to do with that data and find tools to help them really start leveraging that data and that AI technology in the workplace across different industries.
- [Tom] They're already doing aspects of this. And some companies might be 20%, some that might be 90% because they're already using their data for analytics, but it might be traditional analytics, right? So I think the first question is do you understand the data that you have, where it lives, and how to get it into usable forms? And then curated forms. And I think we're going to still have lots of debates over do I just need to make it usable or what's the additional value of going through the curation steps, which can be very time consuming and a lot of costs associated with.
It's really interesting when you start seeing some of the comparisons, which is just give me as much data as you can versus give me the right data with the right curation around it. But I think for organizations, it's if they understand and again, there's a continuum here of maturity, they understand their data, do they have it in a usable form, and then I always like to point back to it's, look, data is just data. It's really about creating better outcomes or as one executive put it to me, it's look, we want to make a better decision today than we did yesterday. We want to consistently make better decisions. We want to make them faster. And then we want to do them at an increasing scale. That's why we've organized our data and how we use it to create competitive advantage. And I really like that definition in the business context because that's really what it is. It's not just about having data or having a great program or using AI. It's about creating a better business outcome for a customer, a more frictionless experience for someone, getting more done in the day, growing your business faster. It ultimately comes down to AI is just the latest tool we've been introduced to that might help us win in whatever business we're in.
- [Neil] I love the way you put that, Tom, that you're really just trying to find a different way and a better way of performing the work. I see that for some people though, their perspective is you're trying to fix something that's not broken. Why are we even trying to meddle around with this? What's your experience with that and how do you like handle those situations?
- [Tom] Yeah, so that's a, I think that's a great example. When you focus on outcomes, if AI, if using some of these new AI tools doesn't create a better outcome, if the customer doesn't get a better answer, or we have it reduce the cycle time, then what's the real benefit of the tool? It's not, right? And that's where, that's, I think that's how you separate the hype from the reality, which is at the end of the day, if you're in a business, it's about the metrics that you set for how to measure the success of that business.
And so I push back a lot on that. It's like so if we do this, what's going to be better? So I think Neil, you and I talked about it together, it's we're trying to do clinical trials matching using these new tools. What's the benefit? Every percentage increase of a clinical trial matching we do with our critically ill patients is hundreds of millions of dollars to our top line. CEO can wrap his head around that concept and say, yeah, I'll support that, put a little bit of money to see if you can move the needle. And if it works, we'll double down on the investment. And I think that's what good business leaders are doing. The question is how much do they understand this technology, or they have people around them who are basically saying here's what we could try to do. And then do you have a format for experimentation? It's let's do this on a small scale and see if we can prove out that a better outcome could be generated. And then if we say yes we can, now we got to figure out how to scale it and then take it over to the next business.
And this is what you see when you sit down with a large, multinational company, or you talk to someone like Blackstone who has hundreds of companies within the portfolio. They're trying to see where the wins are and then figure out how to scale those things as fast as and as broadly as possible.
- [Ryan] Yeah, that seems like good advice for companies of all sizes.
- [Tom] Yeah. That can go across sectors.
- [Ryan] Industries as well, absolutely.
- [Tom] One of my mentors once taught me the future is already here, it's just unevenly distributed. And I, so I'm always looking at so who's already doing this. And again, you go back a generation ago, personalization. Who's the first to really do it? Amazon was actually the first to actually start building personalization by using the data. They built that company essentially as a data platform that was buying and distributing goods. But now everyone knows how to do personalization, right? But they were the early adopters. And so I'm constantly looking for can I find the early adopters in different industries and then bring them back to our context and say it's not so crazy, you know, what we're talking about. We're just not there yet. And if we can get there, we will be the early adopter in our environment.
- [Ryan] Where do you see this all going? Just generally speaking with, obviously there's been things companies struggle with years ago that are now becoming easier to do with data and in the AI space, and then as we get into more 2024 and beyond, is there anywhere that you're seeing current struggles easing up and companies being able to leverage and handle and adopt these technologies and solutions more easily and just what do you see the benefits long term?
- [Tom] Yeah, I do. I think, so I'll use a story that I think I'm the only one old enough here to actually have been through it. So I remember when the internet came in, came into play, and statements were made, and it actually, a colleague reminded me of the statements, like other than exchanging emails, what's the use case for the internet? There was a time when that was actually something that was said. Now, we look back at it, that's ridiculous. Think of all the progress that's come out of the internet, the connectivity, the ability to purchase things, to find information, curated experiences.
And so that's why I say I think this is, that's a great analog because most of us can remember the beginnings of the internet, and I think we're there now, and we're seeing just a very early stage of what these tools will be able to do. They'll be able to augment our intelligence and actually reduce cognitive load, create better outcomes, maybe to help us do things faster with less friction, but I think that's only the beginning. I think we're going to see not just these models get better, but much in the way that, you know, when Uber came into existence, it wasn't one technology. It was the internet. It was GPS availability. It was the smartphone. It was, electronic financial payments. It was the ability to do rating.
You needed to bring different technology components together to create a superior solution that customers wanted. And I think that's what we're going to see here. It's not just about AI or generative AI, it's that plus what immersive experiences are continuing to evolve our ability to do. It's our ability to leverage conversations and experiences in a way that seem almost like, almost human.
I think we're going to see more and more contextualization, which again, I think we're at the very early stages of, contextualization of I ask a question, but based on what you know about me to this moment plus what the large language model behind put together gives me a contextualized answer that I'm looking for and companies that are really smart are going to point me to where they want me to go next, which might be to buy their product or upgrade my certain subscription. And so this is, and they're going to not necessarily need to differentiate between a physical experience and a digital experience. They're going to be much more blended. And again, that might be really difficult for me to wrap my head around, but to a 15 year old today who's 25, they're going to be a phigital individual. They're going to have a physical and a digital persona that are going to be much more blended than mine is, for example.
So we, what I see is that we're just, that's why I say the Tour de France is a great analogy, which is I think we're in early stages, but as we evolve, we're going to see great innovations through creative ideation but by combining technologies in a way. Think about the future media and entertainment. This is going to be a huge disruptive force, not just in terms of the creation, but the distribution, the experiential component. You're going to be able to be in the movie, not just watch the movie. And that's going to be something that people are going to pay for, a premium for in the beginning, and then someone's going to bring it down to an affordability at a mass market level. And the concept of sitting in a movie theater watching a movie is going to be retro.
- [Neil] We're not that far away.
I think it's Xtadium, they have courtside seats to the NBA games. They have two 70 degree angle cameras, and if you have the service, I think they do it in partnership with the NBA, it's like you're sitting in that court side seat watching the game, and you can move your head around and the camera will show you the proper angle. It's crazy. We call that combination of convergence. And we had, we did a previous episode with Lia DiBello, who is a world renowned cognitive scientist, but talking about how she's leveraging AI and the metaverse to effectively teach people creative thinking, critical thinking, improve their risk management experimentation to come up with better decision making. It'll be fascinating to see what the world will be like in 10 years.
- [Tom] It is. Neil, to that example, Walmart has rolled out immersive training for their store managers to help them be better prepared for different scenarios. They saw enough business value, both from the standpoint of scaling training of not having to have people just watch videos or fly them to centers, that they're now using headset based training for store managers.
And if you've ever worked with Walmart, they might be from the Midwest, but they are very technology savvy, and they have incredible scale when they decide that they see something that works. And they're doing this at scale now. And I think it's an incredible use case because it's not a sexy use case. It's a meat and potatoes, we've got X number of tens of thousands of stores and those individuals need to be ready for the challenges that are confronting them each and every day that they go to work. It's a very classic how do we make the organization better story?
- [Neil] I like your example there, like meat and potatoes, because I think too many organizations are looking for the sexy story. I remember when we announced we were going to go into healthcare with AI, I was advocating let's just create a patient intake tool. Let's give doctors an extra five minutes with the patients that way. And it's just no, that's not an exciting enough story. Let's tell people we're going to cure cancer. It's like oh my god. That's, people really think that's going to happen in three months and then when it doesn't happen, they're all going to say this stuff doesn't work. It's all hype. But I think exactly, your meat and potatoes, that's the spots to be looking for those AI opportunities.
- [Tom] Yeah, certainly. I mean look at Amazon, right? A juggernaut of an organization on many levels now. What did they sell? The first thing. Books. Couldn't get more boring. And of course you weren't going to be able to do anything other than books, right? It's like oh someone's going to buy a pair of shoes? Yeah, I mean, again, the paradigm changed, and I think it's one of the things I love about business, and I love about being in this country is we're always looking for the next thing that we can shift people's thinking on. And, but I'll come back to something I know that for Neil and I are very important, which is this has got to be something that's for all of us. And that's going to be a real challenge here, right? Because the really creative people, they're going to go after that 1 percent of market because that's what raises the funding, and that's what creates the valuation, but we got to figure out a way to make sure that this doesn't exacerbate the disparities that we're struggling with in our society.
- [Neil] If people want to keep up with your work, Tom, and see the great stuff that's happening at UC Irvine, what's the best way for people to stay in touch?
- [Tom] Yeah, so LinkedIn is where I do all the cool things that we've got going on, is my main source of the kind of things that we're doing. I also have a podcast that I hold, again, trying to bring people at the intersection of what this technology is impacting in our society and trying to bring thought leaders like yourself into to hear about it. So those are the best ways to watch my journey.
- [Ryan] Yeah, I appreciate it again. Excited to get this out to our audience. As things continue to progress throughout the year, it'd be great to maybe have you back later on this year and talk about things that you've seen, things that we're seeing, and just continue the conversation because it's been a good one.
- [Tom] Neil and Ryan, thank you for having me today. It was fantastic being able to chat with you on this.
Special Guest
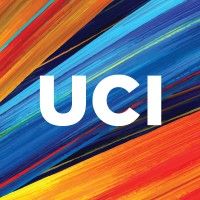
Tom Andriola
- Chief Digital Officer, UC Irvine
Hosted By
AI For All
Subscribe to Our Podcast

YouTube

Apple Podcasts

Google Podcasts

Spotify

Amazon Music

Overcast











