Bringing Generative AI and PLM Together: Where to Start?
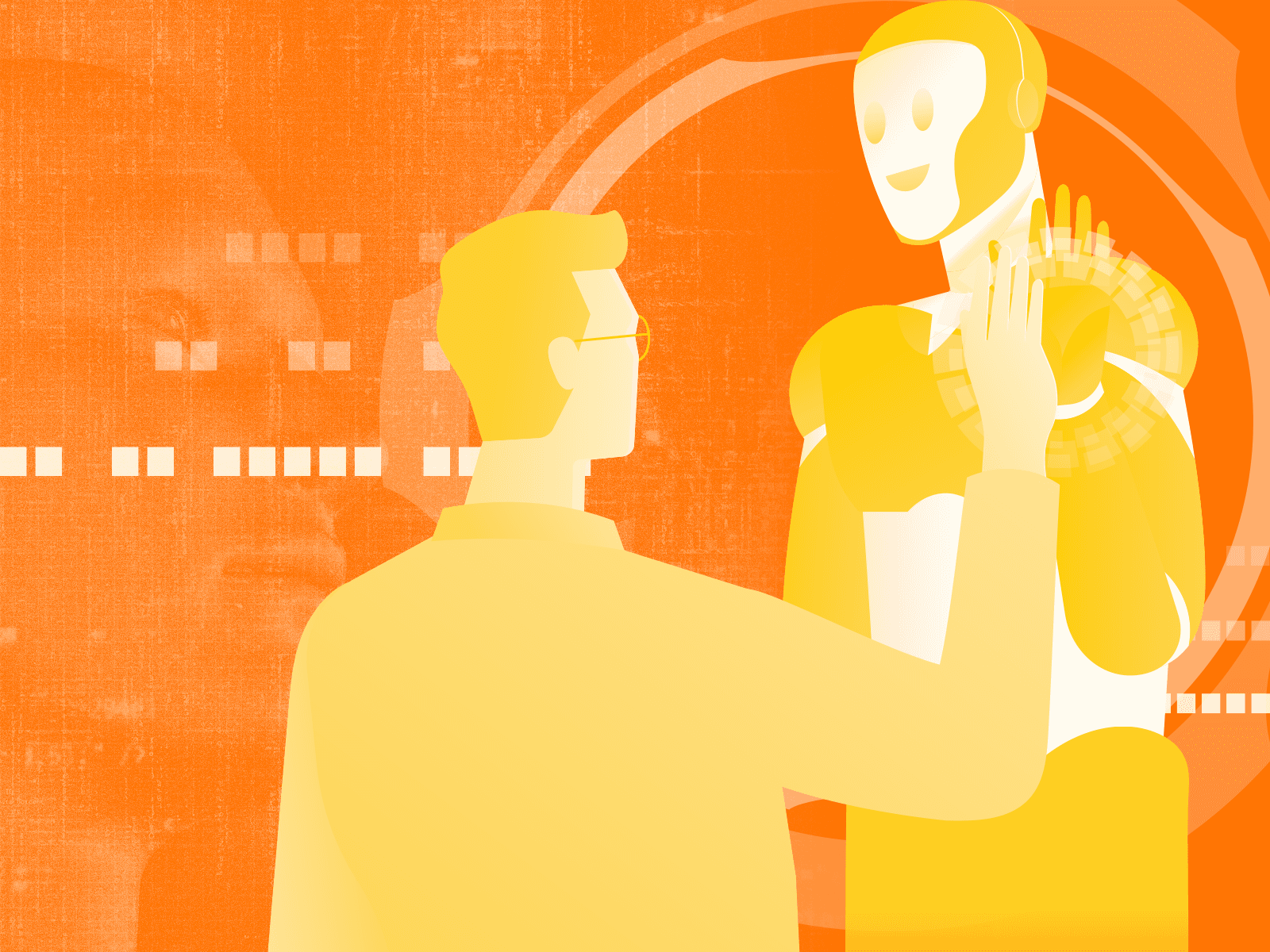
Illustration: © AI For All
In its relatively short time as a mainstream technology, generative artificial intelligence (GenAI) has found a way to gain attention in every industry across the globe. While some uses of GenAI are more immediately useful than others, widespread adoption seems to be a certainty over time.
The product lifecycle management (PLM) space is no exception. Industry analyst Gartner predicts that generative AI will play a role in 70 percent of text- and data-heavy tasks by 2025, up from less than 10 percent in 2023.
They have also concluded that by 2026, generative AI capabilities will be implemented in 50 percent of PLM vendor solutions instead of the current 5 percent adoption rate.
There is tremendous promise in the application of AI throughout the product design and manufacturing process. However, integrating AI also presents some significant challenges for businesses navigating this complex - and quickly evolving - landscape.
The biggest challenge may be to avoid getting swept up in the AI euphoria. There are two big lessons for implementing any new technology. The first is to start small.
Address “low-hanging fruit” - areas where fast time-to-value can be achieved. The second is to make sure that any new technology solves real problems and isn’t just for show.
Where to Apply AI
There are a handful of examples in PLM and product design processes where the application of AI-based tools can make a noticeable difference for companies. It’s best to focus on driving greater efficiency and productivity out of existing processes, rather than attempting to replace work with AI automation.
We are already seeing AI utilized in the form of “copilots” or intelligent, natural-language virtual assistants that can answer questions and guide users to answers through specific procedures.
The idea is to make the user’s job easier and to speed up some of the mundane or time-consuming tasks they have to deal with.
Some examples of the areas where AI will make a difference shortly include:
Compliance
Identifying the correct set of regulatory compliance requirements when designing products is a difficult and often complex task. It intensifies with the number of industries and regions a product is ultimately intended to be sold to or used in.
Failure to accurately consider regulations increases the risk of either overdesign (too many requirements added), or results in a failure to be approved (missed requirements). By incorporating AI into the process, the identification of which requirements - and the ultimate review of a design in the process - can be accelerated.
A global review of regulations and compliance checks can be accomplished faster than ever with the aid of AI. The ability to train the AI to remain up-to-date with the latest regulatory changes can provide ongoing value.
As anyone who’s worked in product design before knows, these can change frequently and with inconsistent notice. They can also cause major headaches if not addressed as a part of the design and approval process.
Technical Documentation
Drafting technical documentation can be a time-consuming process, but it’s critical to the efficient manufacture, testing, and operation of many products. Generative AI can assist in drafting user guides, maintenance manuals, and other important documents.
Technical writers and editors will still have the lead role in ensuring accuracy and completeness, but AI can provide a head start in content generation.
Search and Discovery
An AI-based chatbot can provide engineers the ability to quickly find relevant components and documentation using natural language search. This can both make engineers more efficient and improve designs through increased reuse of existing content.
Moving forward, AI can begin making suggestions for engineers to discover content relevant to their designs. This proactive approach will accelerate adoption and will provide increasingly better feedback over time.
Simulation
The rapid growth of simulation in product development processes has made engineers’ time even more valuable.
Leveraging AI to streamline and optimize simulation workflows holds the promise of getting better results with lower investments of time. If AI can recognize patterns and provide automated alternatives for repetitive tasks, better products will result.
In the future, the possibilities of AI in the manufacturing and product development industry will expand even further.
AI holds the potential to accelerate design cycles, improve product quality, and impact the actual design of organizations’ core product offerings by offering suggestions and improvements along the way. AI technology could also one day be used to drive product innovation by analyzing customer feedback and market trends.
We’re not there yet, but it’s easy to see how AI technology can become a complementary tool for designers and engineers.
Potential Issues
As with any technology, there can be challenges in implementing AI. Deploying AI for PLM and product development scenarios presents a few unique problems.
First, it’s important to understand that PLM tracks some of a company’s most important Intellectual Property (IP) - the designs of its products. Protecting that IP should be paramount, but exposing the data to AI can put it at significant risk. If an AI model is trained on proprietary data, likely, the results will likely also contain proprietary information.
As an extension of the first challenge, even within an organization, there is frequently a need to limit access to particularly sensitive IPs. The staff working on a secretive new design should have access to AI to innovate faster. However, training the AI on this data risks exposing it to others in the organization.
We all know that AI runs on data. AI utilizing high-quality data is likely to return good results, but AI trained on bad data can be dangerous.
In that case, AI would not only spread incorrect information. It would also make bad inferences, which would result in additional bad data. It would create a multiplying effect for data quality issues.
Most importantly, we must recognize that companies depend on PLM to build products that we use in our daily lives. The cars we drive, the elevators we ride, and even the devices we keep in our pockets must be safe for humans at all times.
We can’t depend on AI to only give us correct answers. We must have strict governance processes and verification methods to ensure compliance with all regulations, as well as a keen focus on safety.
Embracing the Future of AI + PLM
The integration of AI into PLM platforms and related functions represents a significant milestone in the evolution of product design and engineering. AI can help improve efficiency, lower costs, and unlock new opportunities for innovation.
Just remember to begin where the value of AI can be realized, managed, and understood by the organization as a whole. And be sure to understand all risks to avoid the pitfalls of misusing this exciting technology.
Generative AI
AI for Business
AI Data
Author
Aras is a leading provider of product lifecycle management and digital thread solutions. Its technology enables the rapid delivery of flexible solutions built on a powerful digital thread backbone and a low-code development platform. Aras’ platform and product lifecycle management applications connect users in all disciplines and functions to critical product data and processes across the lifecycle and throughout the extended supply chain.
Author
Aras is a leading provider of product lifecycle management and digital thread solutions. Its technology enables the rapid delivery of flexible solutions built on a powerful digital thread backbone and a low-code development platform. Aras’ platform and product lifecycle management applications connect users in all disciplines and functions to critical product data and processes across the lifecycle and throughout the extended supply chain.